The E.YU Lab integrates multidisciplinary approaches that encompass epidemiology, health statistics & bioinformatics, multi-omic techniques, wet-lab experiments, and clinical practice to combat the development and progression of complex traits.
Live well in TREND
We initiated the TRacing Etiology of Non-communicable Diseases (TREND) cohort with Prof. Lianmin Chen. This prospective longitudinal cohort and biobank is mainly based in Ma’anshan, Anhui Province, China. The study’s primary aim is to decipher comprehensive molecular characterization and deep phenotyping for a broad spectrum of chronic non-communicable diseases (NCDs), which focuses on providing mechanistic insights with diagnostic, prognostic, and therapeutic implications.
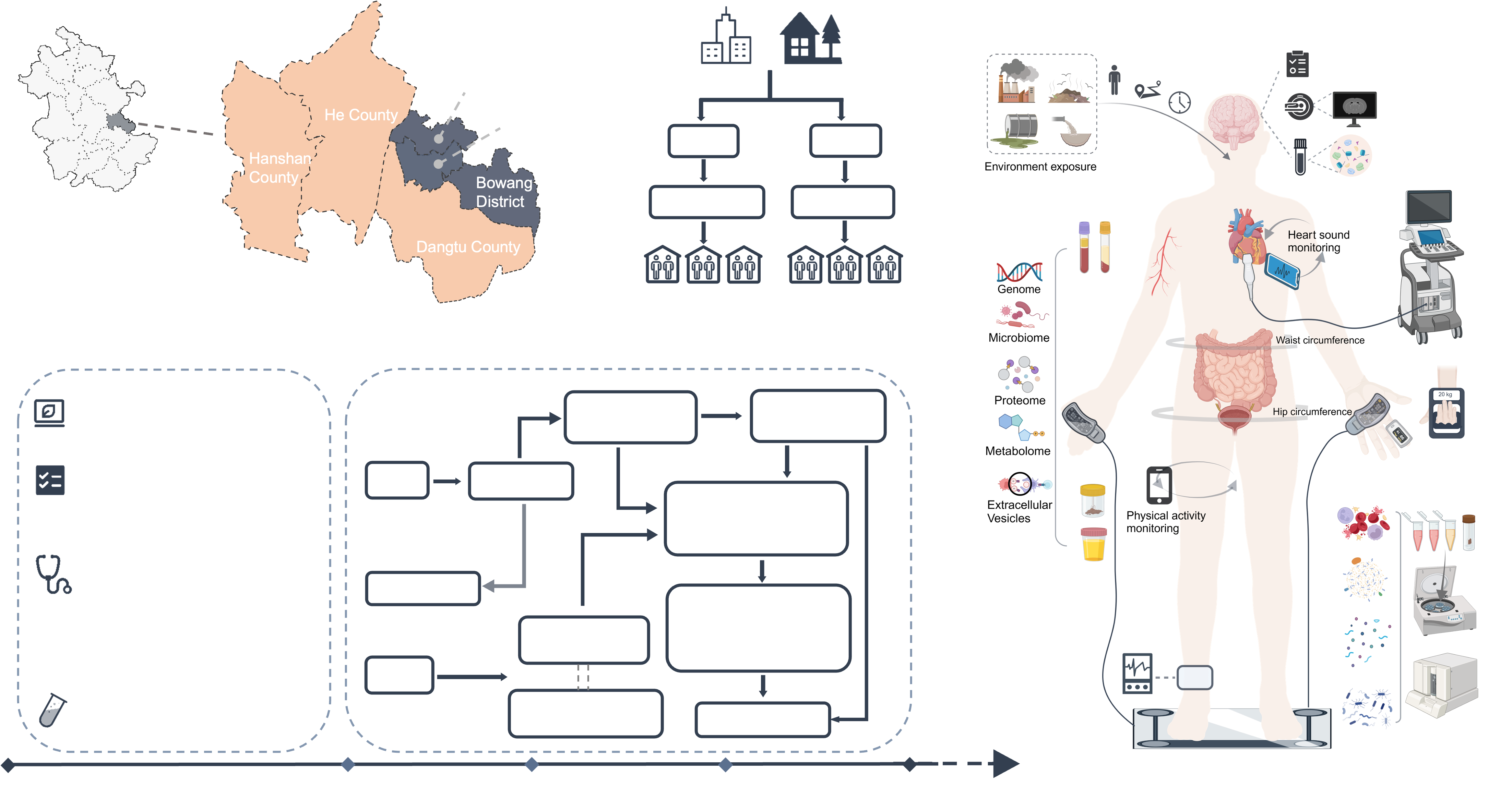
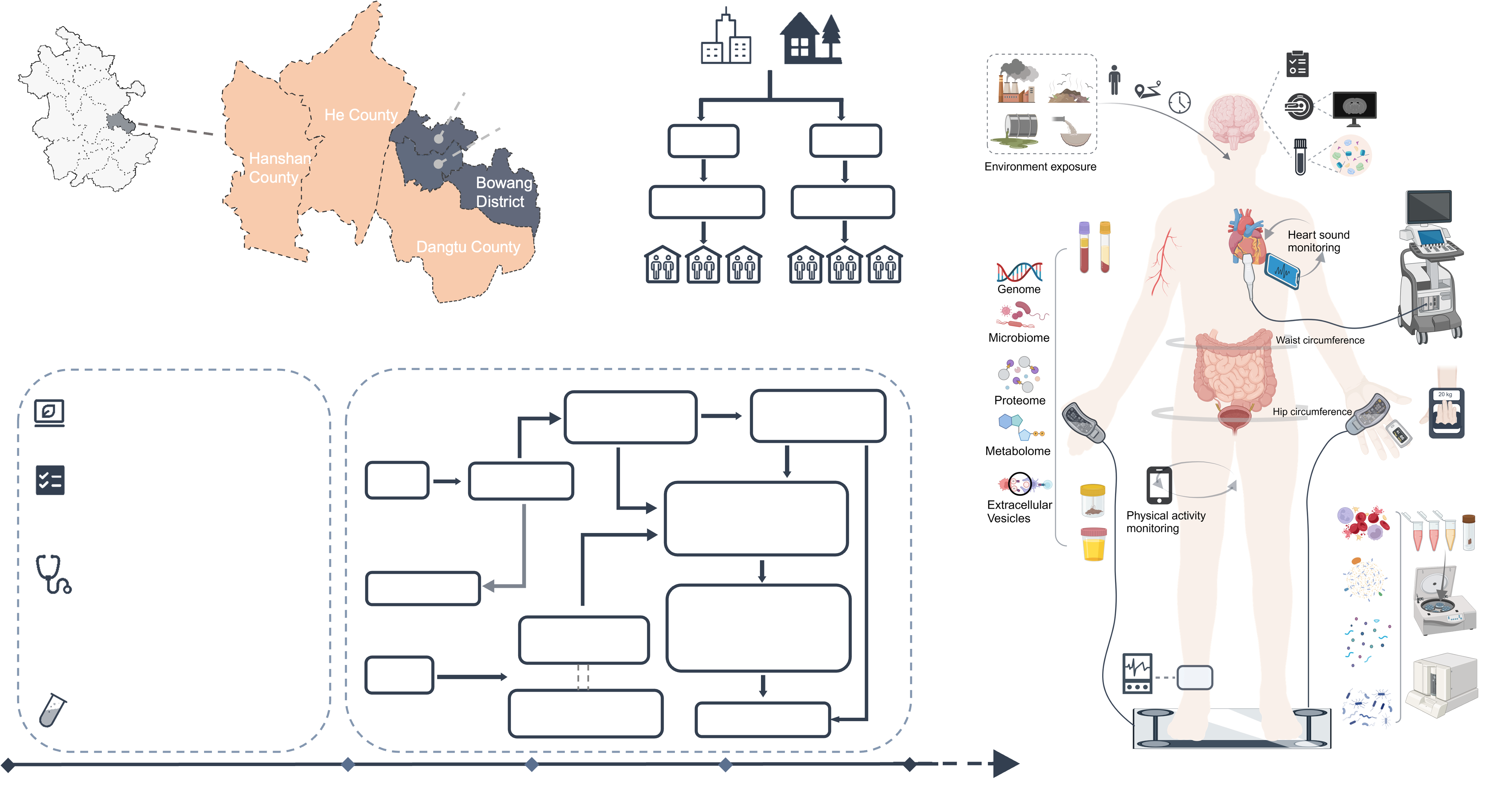
We collaborated with Dr. Anke Wesselius on the Maastricht Study, an extensive phenotyping study focusing on the etiology of type 2 diabetes. We collaborated with Prof. Maurice P. Zeegers on the Bladder Cancer Prognosis Programme (BCPP) and the Bladder Cancer Epidemiology and Nutritional Determinants (BLEND) international consortium, aiming to provide a unique resource for a variety of bladder cancer studies. We also initiated our urinary-cancer-based cohort at Southeast University, investigating the etiological and molecular characterization of prostate, bladder, and renal cancers. We integrated the whole exome sequence and high-throughput MS-based detection of human molecules at the world’s largest center for hyperlipidemia pancreatitis populations to demonstrate the beyond molecular trajectories and therapeutic targets. We also have accessed to some publicly available datasets, such as UK Biobank, NHANES, CHARLS, etc.
Build-up "LEGO" of Omics
Similar environmental and lifestyle patterns may cause different health effects in different populations. A key aim of modern-epidemiological research therefore is to determine the role of molecular landscape in regulation and to identify factors that influences an individual’s response to environment and lifestyle. Multiple new opportunities regarding this aim have recently been created by remarkable advances in omics technologies, including genomics, metabolomics, proteomics, gut microbiome, etc.. These novel omics technologies hold great potential to get deeper insights in the complex relation between environmental/lifestyle and chronic diseases. Collaborating with EVLIXIR, we have developed our in-house pipeline for certain hidden and emerging characters, e.g., extracellular vesicles (EVs). In the sooner future, our lab would take efforts to dig in deeper, mainly based on extracellular vesicles, for revealing novel insights of EVs in human health.
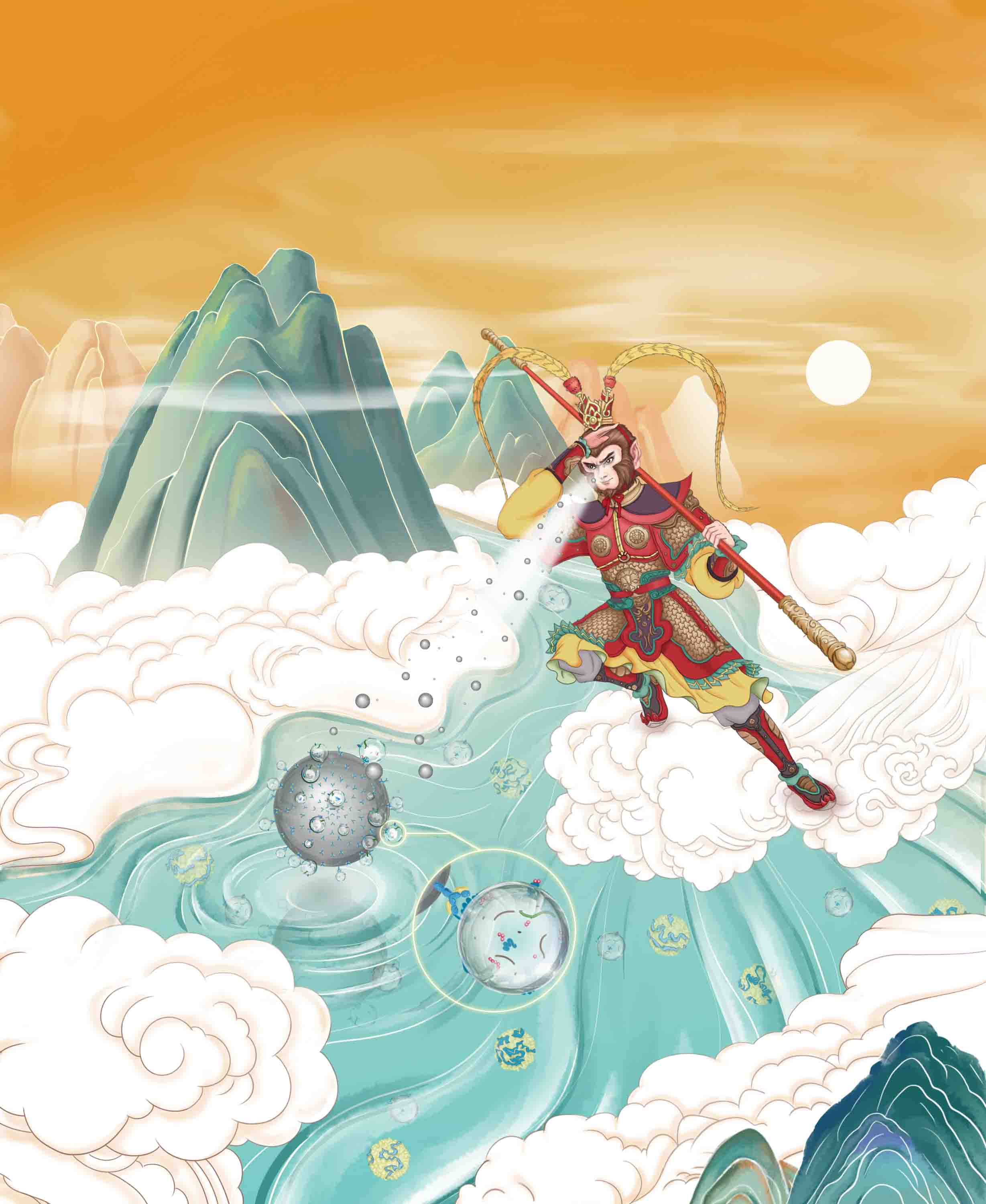
Personalized monitoring of YOU
Future of tailored healthcare and technology, where data is collected and analyzed to provide a customized experience. By tracking vital metrics such as heart rate, glycaemic dynamics (feat. Prof. Jusheng Zheng) sleep patterns, and activity levels, this system adapts to your unique needs. It allows for real-time insights, ensuring that every decision made about your health and lifestyle is based on the most relevant and up-to-date information. Hence, we originally developed and applied Smartphone-Measured Heart Sounds for Screening Cardiovascular Health, collaborating with Dr. Hongxing Luo, for the first time around the word.
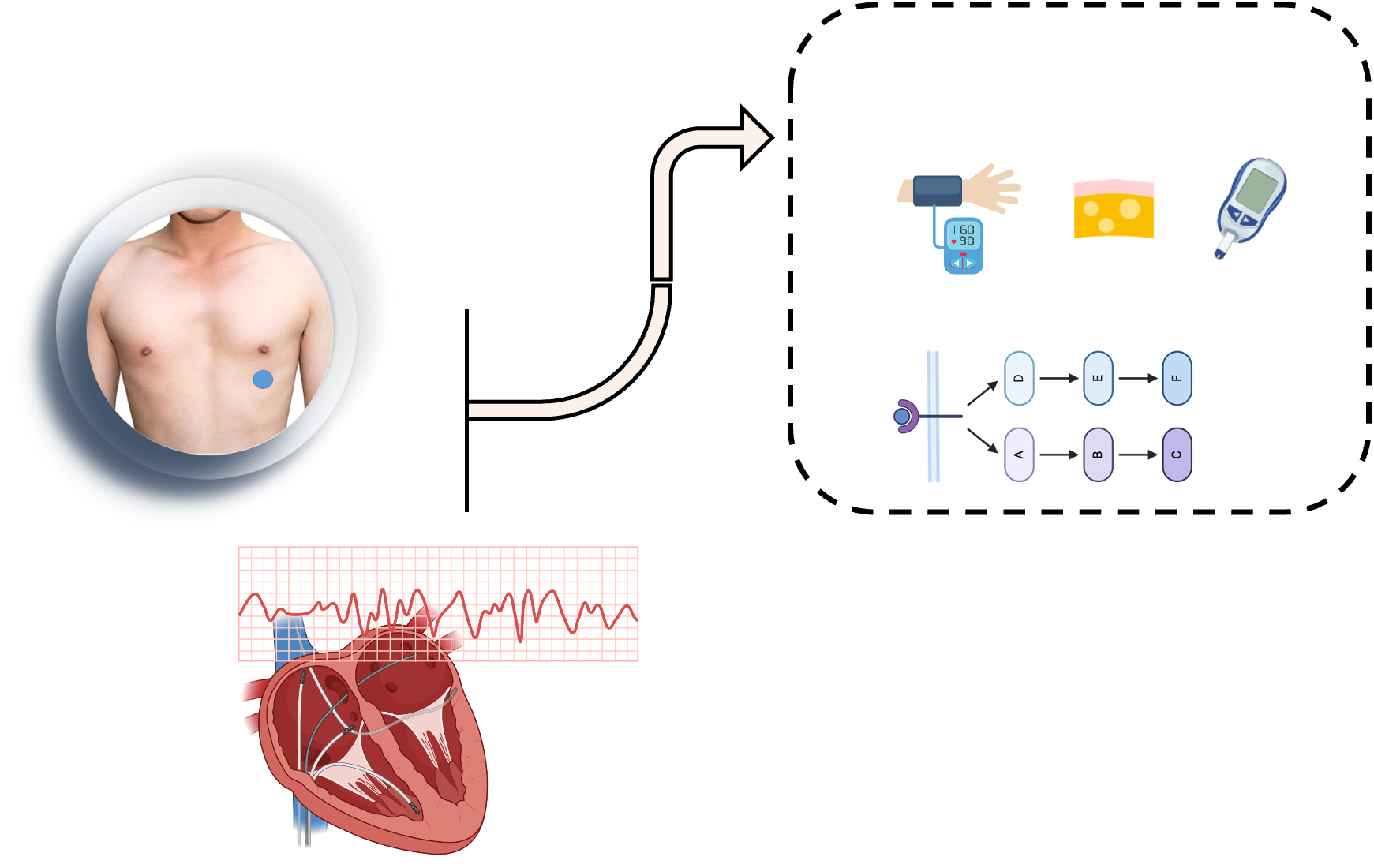
With those personalized approaches, you can optimize your well-being and make informed choices to enhance your daily life. This innovative monitoring system empowers YOU to take control of your health journey, creating a more proactive and personalized experience.
Novel and advanced analysis approach
With the rise of the omics data, epidemiology is entering the "big data" era, as such, the potential for advanced analysis grows significantly. The use of traditional regression and/or survival analysis will likely be replaced by machine learning techniques. Particularly in contexts where prediction or hypothesis generation rather than hypothesis testing is the analytic goal. Machine learning technique applied in the field of epidemiology could help to identifying patterns that relate variables to diseases and maximize accuracy when predicting those outcomes. In addition, algorithmic identification of patterns/items associated with a disease of interest allows epidemiologists to focus on independent validation and interpretation of these associations in subsequent studies.
Therefore, our lab will integrate relative new approaches of hypothesis generation,yet in a very early stage, which enable epidemiologists to move to analysing more complex notions and characterizing how the quantity and timing of exposure influence small molecular weight cellular constituents. However, although the use of big data in epidemiology calls for new skills and collaboration between different research fields (i.e.,knowledge engineering), traditional epidemiological and expert skills will not be removed due to their bona fide multidisciplinary features in understanding how political, social and scientific factors intersect to disease risk.